In today’s rapidly evolving financial landscape, the integration of Artificial Intelligence (AI) is transforming how data is analyzed and utilized. Natural Language Processing (NLP) in finance is at the forefront of this transformation, enabling machines to interpret and manage human language. By harnessing the power of NLP, financial institutions can unlock valuable insights from unstructured data, such as news articles, social media posts, and financial reports.
Introduction
NLP applications in financial analysis are diverse and impactful. Some key applications include automated document classification, information extraction, sentiment analysis of news articles and social media posts, risk assessment, fraud detection, and chatbots for customer service in banking and finance. These tools allow financial professionals to gain a comprehensive understanding of market dynamics and make informed decisions, enhancing both speed and accuracy.
As the financial industry continues to digitize, the role of NLP is becoming increasingly vital, enabling institutions to stay competitive and responsive in a data-driven world. By processing vast amounts of textual data, NLP helps investors and analysts identify trends, assess risks, and make more informed investment decisions.
Understanding NLP and Its Components
Natural Language Processing in finance is transforming how professionals extract actionable insights from unstructured data. The key components of NLP in finance include entity extraction, tokenization, stemming and lemmatization, part-of-speech tagging, named entity recognition (NER), and sentiment analysis.
Entity extraction identifies and extracts entities like companies, people, and financial instruments from text, such as extracting company names and financial figures from earnings call transcripts. Tokenization breaks down text into smaller units, enabling analysis of individual words or phrases in financial documents. Stemming and lemmatization reduce words to their root forms, improving data normalization, which is particularly useful when analyzing variations of financial terms.
Machine learning plays a crucial role in enhancing NLP capabilities. Supervised learning is used for tasks like sentiment classification and named entity recognition in financial texts. Unsupervised learning helps in topic modeling and clustering of financial documents, while deep learning enables more advanced NLP tasks, such as language translation and complex sentiment analysis.
NLP in Financial Document Analysis
NLP has emerged as a transformative force in financial document analysis, particularly in analyzing SEC filings, earnings call transcripts, and news and social media content.
For SEC filings, NLP tools can extract key information from 10-K and 10-Q reports. They can automatically identify and categorize risk factors mentioned in annual reports, extract and compare financial metrics across multiple reports, and ensure all required sections are present and properly formatted.
In earnings call transcript analysis, NLP can provide valuable insights by gauging the overall tone of management’s statements, identifying the most discussed topics during the call, and analyzing how management responds to analyst questions.
For news and social media analysis, NLP tools can process vast amounts of content to identify significant events that may impact financial markets, monitor changes in market sentiment over time, and detect emerging trends in specific industries or companies.
Sentiment Analysis in Finance
Sentiment analysis, a key application of NLP in finance, plays a crucial role in understanding market dynamics by analyzing the emotional tone of various textual sources. Its applications include stock market prediction, risk management, brand monitoring, and developing trading strategies based on sentiment indicators.
However, financial sentiment analysis faces several challenges. Financial texts often contain sarcasm or require contextual understanding, which can be challenging for NLP models. The domain-specific language, including financial jargon and technical terms, can complicate sentiment analysis. Additionally, distinguishing between short-term reactions and long-term sentiment trends presents another challenge.
Advanced techniques in sentiment analysis include fine-grained sentiment analysis, which goes beyond positive/negative classifications to detect more nuanced emotions, aspect-based sentiment analysis, which analyzes sentiment towards specific aspects of a financial entity, and multi-modal sentiment analysis, which combines text analysis with other data sources, such as stock prices or trading volumes.
Benefits of NLP in Financial Analysis
NLP offers numerous advantages in financial analysis, including efficiency, objectivity, real-time analysis capabilities, scalability, pattern recognition, and multilingual capabilities. It automates the processing of vast amounts of textual data, saving time and resources while reducing human bias in data interpretation. NLP enables quick analysis of breaking news and market sentiment, processes large volumes of data consistently and accurately, and identifies trends and patterns that might be missed by human analysts. Its multilingual capabilities facilitate global market analysis by analyzing financial texts in multiple languages.
Challenges and Limitations of NLP in Finance
While NLP offers significant benefits in financial analysis, it also faces several challenges. Data quality and consistency issues arise from the varying formats and quality of financial texts, making consistent analysis challenging. The domain-specific language of finance, including jargon and technical terms, can be difficult for general NLP models to interpret accurately.
Ensuring NLP applications comply with financial regulations and data privacy laws presents another challenge. The “black box” nature of some advanced NLP models can be problematic in finance, where decisions need to be explainable. Financial texts often require contextual understanding and can be ambiguous, necessitating more sophisticated context-aware NLP models. Additionally, the rapid evolution of financial markets requires constant model updates to keep up with changing dynamics.
To address these challenges, solutions include implementing robust data preprocessing and cleaning techniques, developing domain-specific NLP models trained on financial corpora, implementing strict data governance policies and regular compliance audits, focusing on developing interpretable models and using explainable AI techniques, and implementing continuous learning systems with regular model retraining.
Future of NLP in Financial Analysis
The future of NLP in finance looks promising, with several emerging trends and potential developments. These include more advanced sentiment analysis techniques, multimodal analysis combining text with other data types, the development of more interpretable NLP models, real-time analysis enabling automated trading decisions, personalized financial advice through NLP-powered chatbots and robo-advisors, more sophisticated fraud detection and risk management models, and improved capabilities in cross-lingual financial analysis.
As NLP becomes more prevalent in finance, ethical considerations will become increasingly important. Ensuring fairness and avoiding bias in NLP models, balancing the need for data with individual privacy rights, and providing transparency in NLP-driven decision-making processes will be crucial challenges to address.
In conclusion, NLP is revolutionizing financial analysis by enabling more efficient, accurate, and insightful processing of textual data. While challenges remain, the continued development of NLP technologies promises to further transform the landscape of finance, empowering professionals with unprecedented analytical capabilities and insights.
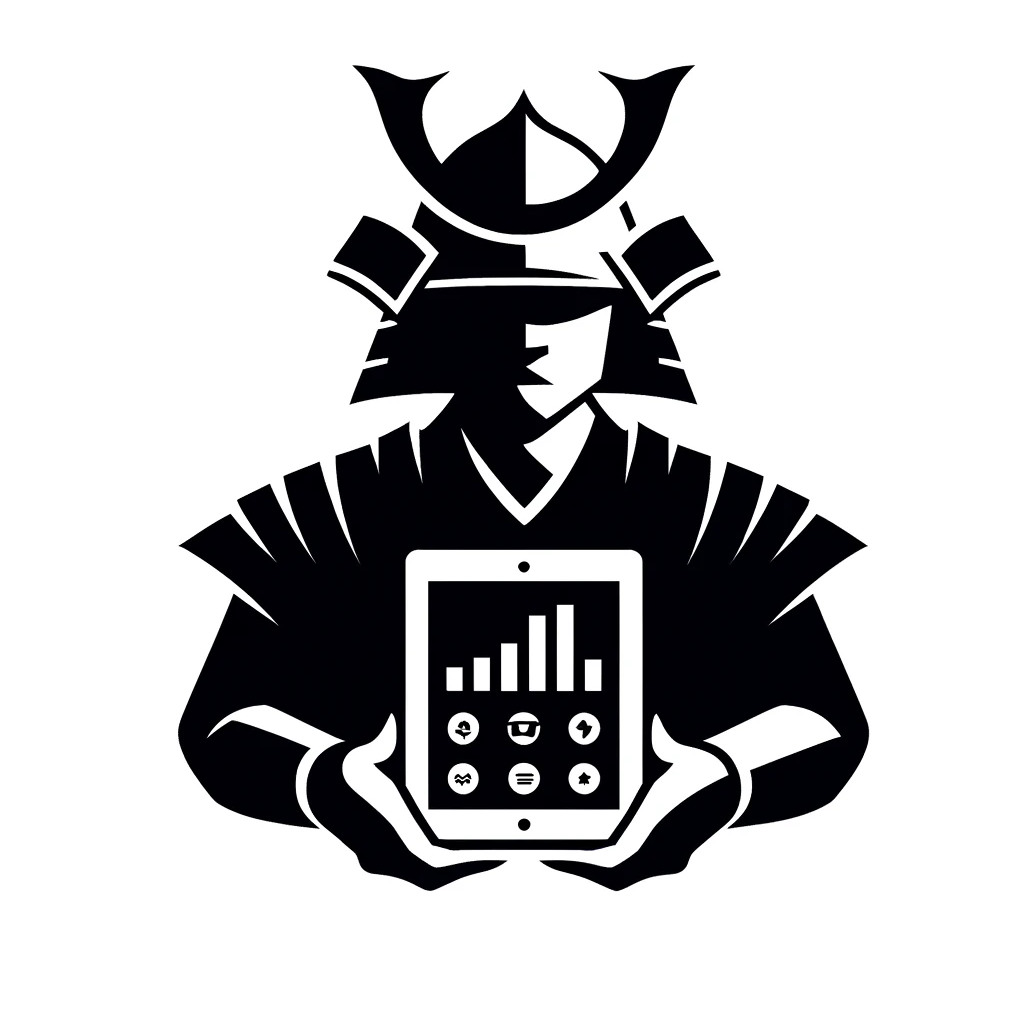